The Ultimate Guide to Video Labeling Tool Machine Learning
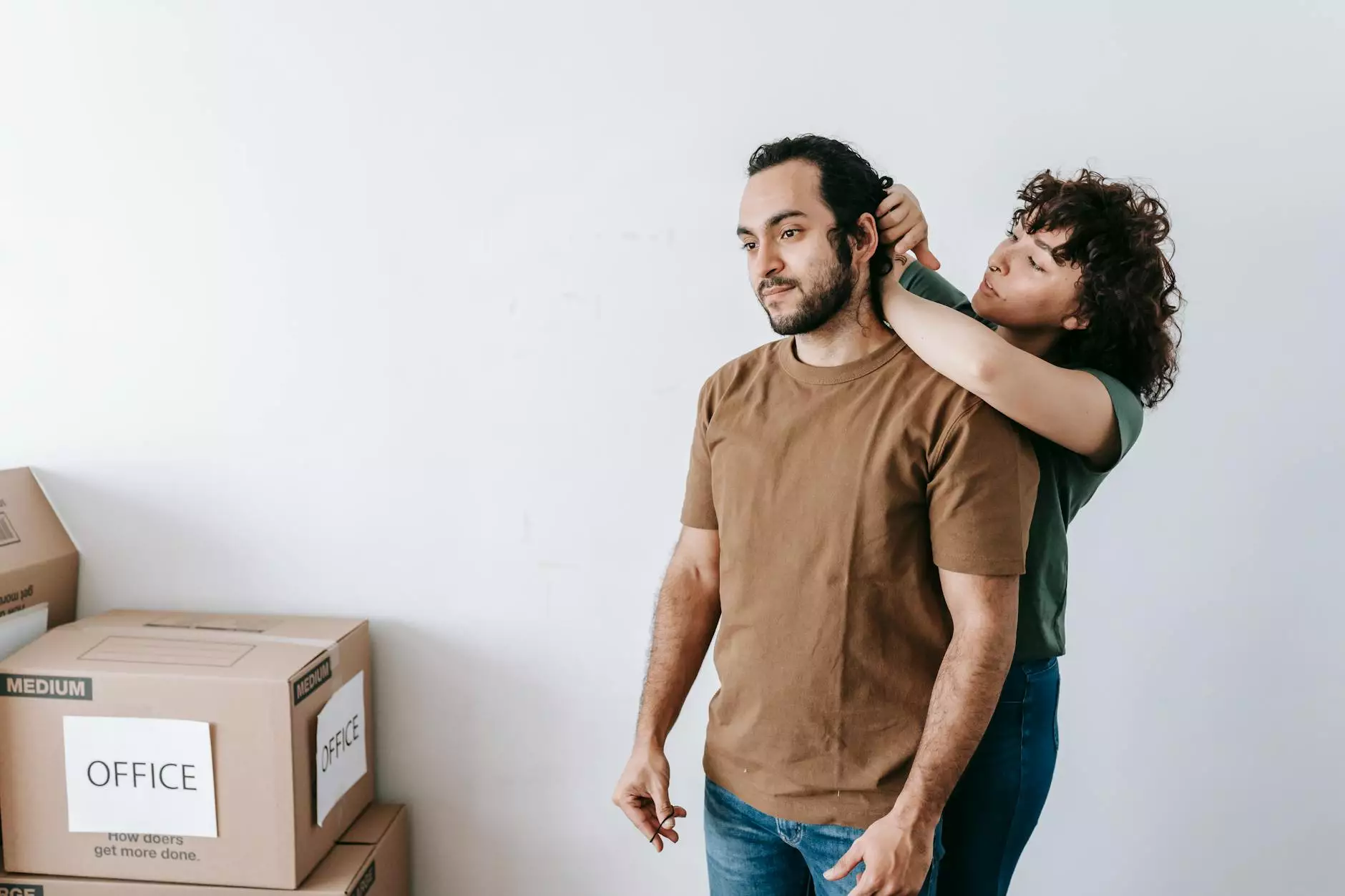
In the rapidly evolving landscape of artificial intelligence (AI) and machine learning (ML), the importance of precise and effective data annotation cannot be overstated. Businesses across various sectors are recognizing the value of tools that simplify and enhance the data labeling process, particularly when it comes to video content. This comprehensive guide focuses on the significance of a video labeling tool machine learning framework and how platforms like Keylabs.ai are revolutionizing data annotation.
Understanding Video Labeling in Machine Learning
Video labeling involves the process of annotating video data, which is crucial for training machine learning algorithms. By providing accurate labels, businesses can ensure that their AI models learn effectively, thereby enabling them to make precise predictions and insights. This process is vital in various industries, including:
- Healthcare: Used for diagnosing conditions through video analysis.
- Autonomous Vehicles: Essential for understanding and interpreting the environment.
- Security: Important for identifying suspicious activities through surveillance footage.
- Sports Analytics: Used for performance analysis and strategy formulation.
Why Invest in Video Labeling Tools?
Investing in a video labeling tool machine learning is a strategic move for businesses looking to harness the power of AI. Here are several reasons to consider:
- Improved Accuracy: Machine learning thrives on quality data. Accurate annotations result in better model performance.
- Time Efficiency: Automated labeling tools significantly reduce the time required for data preparation.
- Scalability: As video data grows, the ability to scale annotation efforts becomes crucial.
- Enhanced Insights: Properly labeled video data can reveal trends and patterns that drive business decisions.
Key Features of Video Labeling Tools
When choosing a video labeling tool, businesses should consider the following key features:
1. User-Friendly Interface
A well-designed interface can make the annotation process intuitive and efficient. Look for tools that allow for easy navigation and quick access to labeling functions.
2. Support for Multiple Video Formats
With the diversity of video formats available, a good labeling tool must support various formats to cater to different project needs.
3. Collaborative Features
Collaboration among team members can enhance productivity. Features that allow for comments, tags, and shared projects can facilitate better teamwork.
4. Customization Options
Different projects have different needs. Customizable labeling options allow teams to annotate data in a way that best suits their specific goals.
5. Integration Capabilities
The ability to integrate with existing workflows and tools is essential for streamlining the data pipeline from annotation to model training.
The Role of Machine Learning in Video Labeling
Machine learning plays a pivotal role in enhancing the efficacy of video labeling tools. By incorporating ML algorithms, these tools can automate repetitive tasks, suggest annotations, and even predict labeling outcomes. The result? A more efficient and effective workflow. Here’s how machine learning enhances video labeling:
1. Automation of Repetitive Tasks
Automated labeling can significantly reduce the time spent on mundane tasks. Machine learning models can learn from existing data to automatically label new video segments, allowing annotators to focus on more complex cases.
2. Improved Accuracy Through Learning
As more data gets labeled and fed back into the system, the machine learning models continuously improve in both accuracy and speed. This iterative learning process ensures that future annotations benefit from past experiences.
3. Real-time Suggestions
Machine learning algorithms can provide real-time suggestions for annotations based on context, enhancing the speed of the labeling process without sacrificing quality.
4. Enhanced Quality Control
ML tools often include quality control mechanisms that check for consistency and accuracy in labels, which is crucial for maintaining high data quality standards.
How Keylabs.ai Transforms Video Labeling
Keylabs.ai is at the forefront of the data annotation platform revolution, offering advanced solutions in the realm of video labeling. Here’s how Keylabs.ai stands out from the competition:
1. Cutting-Edge Technology
Keylabs.ai utilizes the latest advancements in artificial intelligence to offer powerful video labeling tools that adapt to the needs of various industries.
2. Comprehensive Support
The platform provides extensive support for businesses throughout the labeling process, ensuring smooth implementation and ongoing assistance.
3. Focus on Quality Assurance
Keylabs.ai places a strong emphasis on quality assurance, providing both automated and manual oversight to ensure the highest data quality.
4. Flexible Pricing Models
With flexible pricing models, Keylabs.ai accommodates businesses of all sizes, making it a viable choice for startups and large enterprises alike.
Best Practices for Effective Video Labeling
To maximize the benefits of a video labeling tool machine learning, businesses should adhere to best practices that can enhance the overall quality and efficiency of their annotation processes:
1. Define Clear Guidelines
Before starting the labeling process, define clear guidelines for annotators. This ensures consistency and accuracy in the annotations.
2. Train Your Team
Invest time in training your team on how to use the labeling tool effectively. Familiarity with the tool’s functionalities will lead to higher productivity and fewer errors.
3. Regular Reviews and Feedback
Implement a system for regular reviews and feedback on labeled data. This can help identify areas for improvement and reinforce best practices among annotators.
4. Use ML Features Wisely
Make strategic use of machine learning features in your labeling tool. For example, leverage automated suggestions to speed up the process while retaining quality.
5. Performance Metrics
Establish and track performance metrics to evaluate the effectiveness of your video labeling processes. Metrics such as annotation speed, accuracy, and team productivity can guide future improvements.
Conclusion
The integration of a video labeling tool machine learning approach is essential for businesses looking to harness the power of data in today’s AI-driven landscape. Tools like Keylabs.ai empower organizations to efficiently annotate video content, enabling them to develop sophisticated AI models that bring actionable insights and substantial value. With the right strategies and technologies in place, businesses can tap into the transformative capabilities of video labeling, paving the way toward innovation and success.
Get Started with Keylabs.ai Today!
To revolutionize your data annotation processes and stay ahead of the competition, explore what Keylabs.ai has to offer by visiting keylabs.ai. Unlock the potential of your data and propel your business into the future of machine learning.